Ryosuke Kaneko1,2, Hiromichi Nagao1,2, Shin-ichi Ito1,2, Hiroshi Tsuruoka1, and Kazushige Obara1
1: Earthquake Research Institute, The University of Tokyo
2: Graduate School of Information Science and Technology, The University of Tokyo
Low-frequency tremors, a type of slow earthquake, were discovered in southwest Japan owing to the establishment of spatially-dense seismic observation networks including the High Sensitivity Seismograph Network (Hi-net) in Japan (Obara, 2002) after the 1995 Hyogo-ken Nambu Earthquake. These tremors characteristically have much smaller amplitudes and longer durations —sometimes exceeding several hours— than ordinary earthquakes, and have occurred several times a month on average in southwest Japan since 2001. Tremors are considered to occur along the boundary between the Philippine Sea Plate and the upper plate in areas slightly deeper or shallower than ordinary earthquakes, and are therefore expected to be associated with massive plate boundary earthquakes. Current tremor catalogs (e.g., Obara et al., 2010), which list tremor occurrence times and hypocenter locations, contain only tremor events after 2001. This is because prior to 2001,the seismic observation network was not sufficiently dense to detect tremors using continuous waveforms digitally recorded at multiple stations, a necessary approach to confidently identify tremors. Considering that plate boundary earthquakes in the Nankai trough periodically occur with an interval of approximately 100 or 200 years, it is important to catalog tremors recorded in historical seismograms before the establishment of the modern seismic observation networks to allow a more complete investigation of these earthquake cycles.
In this study, we developed an artificial intelligence technique to detect tremors in a large amount of historical seismograms recorded at the Wakayama Observatory Network of the Earthquake Research Institute, The University of Tokyo, which was in operation about 50 years ago (Fig. 1). Seismographs of that time drew day-long waveforms directly on drum-rolled paper using a pen. A database of scanned images of these historical paper records has been recently developed (Satake et al., 2020). We employed a convolutional neural network based on a residual network (ResNet), and trained it with synthetic waveform images (Kaneko et al., 2021) and more than 50,000 waveform images generated from authentic modern Hi-net digital data. The latter images are meant to imitate the historical seismogram paper records, with the added benefit of knowing when tremors occurred.
The trained network was applied to historical seismograms recorded from 1966 to 1977 by the Kumano station of the Wakayama Network, and successfully identified many previously unknown tremors in the past (Fig. 2). However, we also found some peculiar quirks in the historical paper records that often made tremor detection difficult. For example, due to the technology of the time, it was very difficult for even the most skilled technicians to produce paper recordings plotted with consistent thickness. To solve these challenges and allow the technique to be applied to historical seismograms added to the database in the future, training with a much larger data set is necessary. To this end, we have purchased a state-of-the-art GPU computer for deep learning with the support of the project “Seimology Toward Research Innovation with Data of Earthquake” (STAR-E Project) promoted by the Ministry of Education, Culture, Sports, Science and Technology (MEXT), Japan.
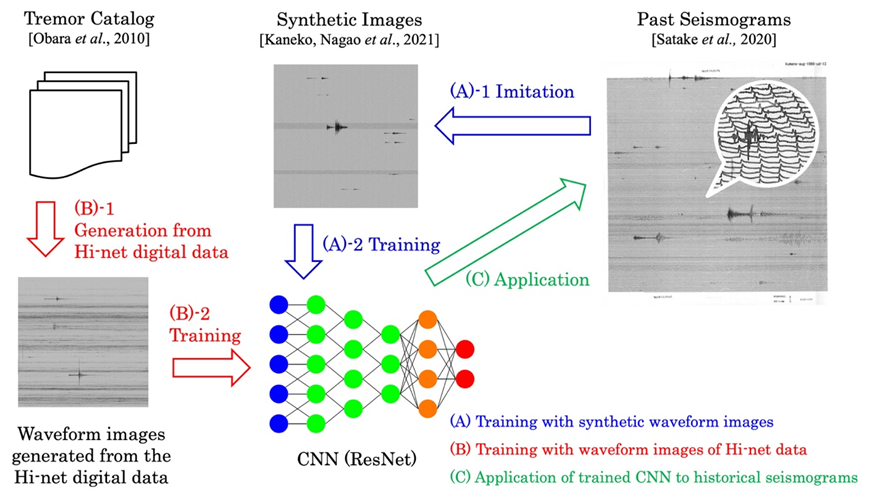
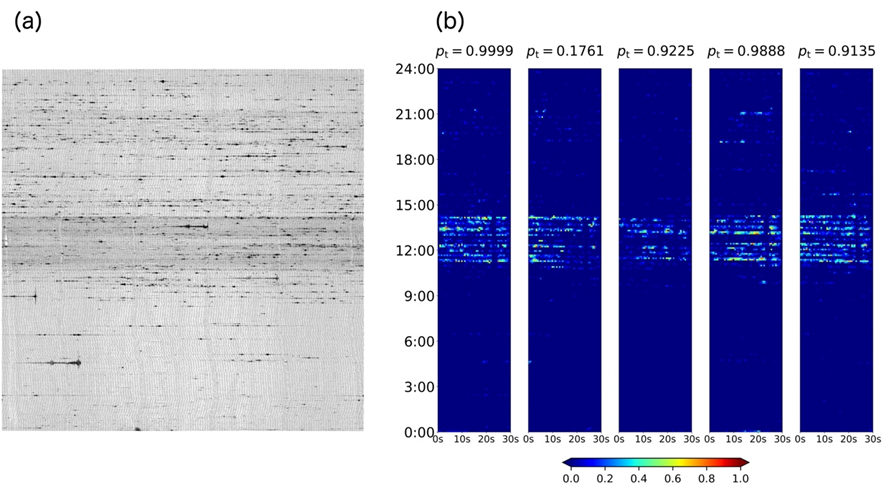
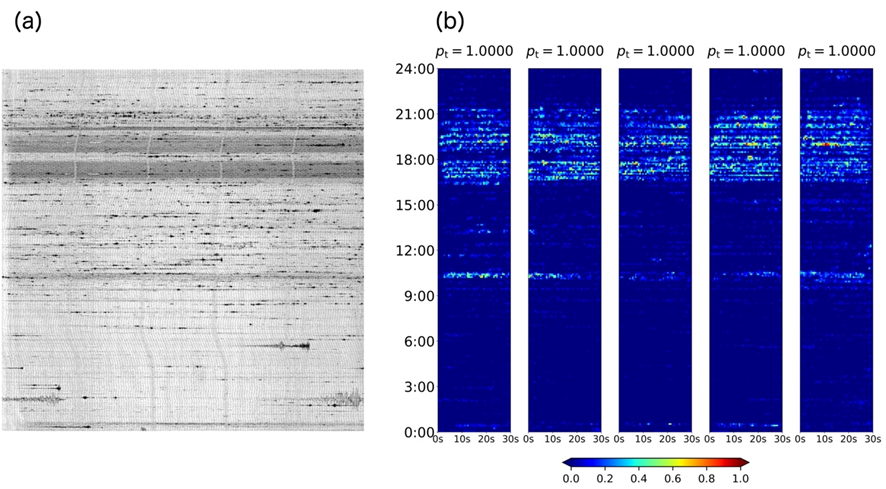